The e-commerce landscape is rapidly evolving, and affiliate marketing is at its forefront. With the advent of machine learning (ML), predicting trends in this dynamic sector has become more accurate and efficient. Machine learning has revolutionized the way businesses operate, particularly in the realm of e-commerce and affiliate marketing.
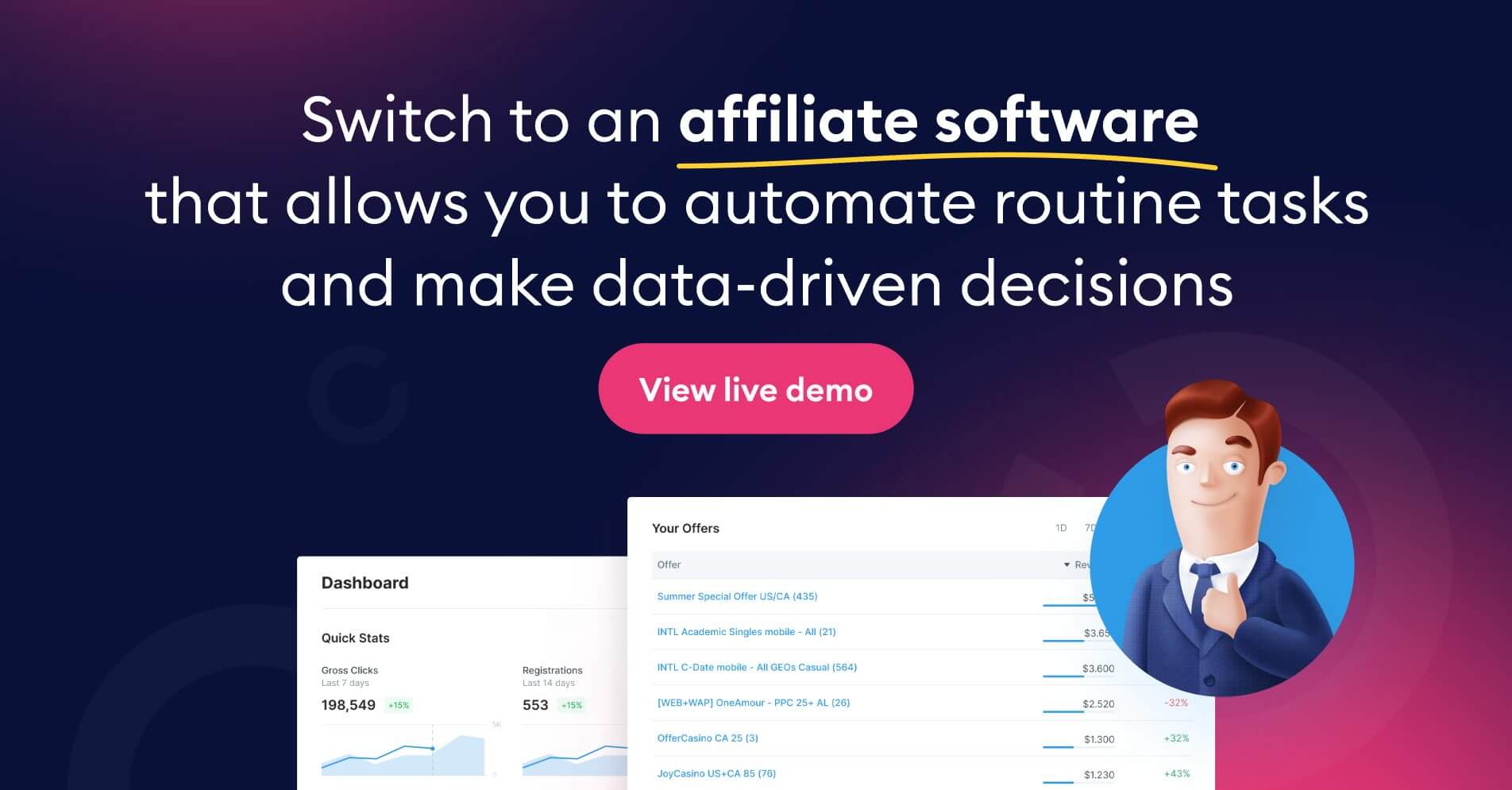
This blog post delves into how machine learning is revolutionizing affiliate marketing for e-commerce businesses.
So, let’s get started!
Understanding Machine Learning in E-commerce
Machine learning algorithms analyze large sets of data to identify patterns, trends, and anomalies.
Here’s a table showcasing how machine learning can be used to predict affiliate marketing trends in e-commerce:
Application of Machine Learning | Function | Impact on Affiliate Marketing |
---|---|---|
Predictive Analytics for Consumer Behavior | Analyzes historical data to predict future buying behaviors. | Enables affiliates to target consumers more effectively with personalized offers. |
Market Trend Analysis | Identifies patterns and trends in market data. | Helps affiliates adapt to changing market demands and preferences. |
Optimizing Affiliate Recommendations | Recommends the most effective affiliate products to promote. | Increases the likelihood of successful conversions and higher revenue. |
Sentiment Analysis of Customer Reviews | Evaluates customer opinions and feedback on products/services. | Guides affiliates towards products with higher customer satisfaction, enhancing promotional strategies. |
Customer Lifetime Value Prediction | Forecasts the potential revenue a customer can generate over time. | Assists in identifying and focusing on high-value customers for long-term gains. |
Personalization of Marketing Campaigns | Customizes marketing content based on consumer data analysis. | Enhances customer engagement and conversion rates through tailored content. |
Churn Rate Prediction | Predicts the likelihood of customers/affiliates discontinuing service. | Helps in taking proactive measures to retain valuable affiliates and customers. |
Fraud Detection and Prevention | Identifies patterns indicative of fraudulent activity. | Protects affiliate revenue and maintains the integrity of marketing efforts. |
Optimization of Affiliate Placement | Determines the most effective placements for affiliate links. | Increases click-through and conversion rates by strategically placing affiliate content. |
Demand Forecasting | Predicts future product demand based on various market indicators. | Allows affiliates to focus on promoting products with higher expected demand. |
Machine learning in affiliate marketing offers a dynamic approach to understanding and predicting market trends, consumer behavior, and the effectiveness of different affiliate strategies. This results in more informed decisions, targeted marketing efforts, and potentially higher returns on investment.
In the context of e-commerce, these algorithms can sift through vast amounts of consumer data to uncover valuable insights related to purchasing behavior, product preferences, and market trends.
Transforming Data into Insights
E-commerce businesses generate enormous data daily, ranging from customer behavior to sales performance. Machine learning algorithms process this data, transforming it into actionable insights. These insights help affiliate marketers to make data-driven decisions, optimizing their strategies for better results.
Leveraging Data for Predictive Analysis
By harnessing machine learning for predictive analysis, e-commerce businesses can anticipate upcoming trends in affiliate marketing. These insights enable companies to allocate resources efficiently, refine their marketing strategies, and stay ahead of the competition.
Benefits of Machine Learning in Affiliate Marketing
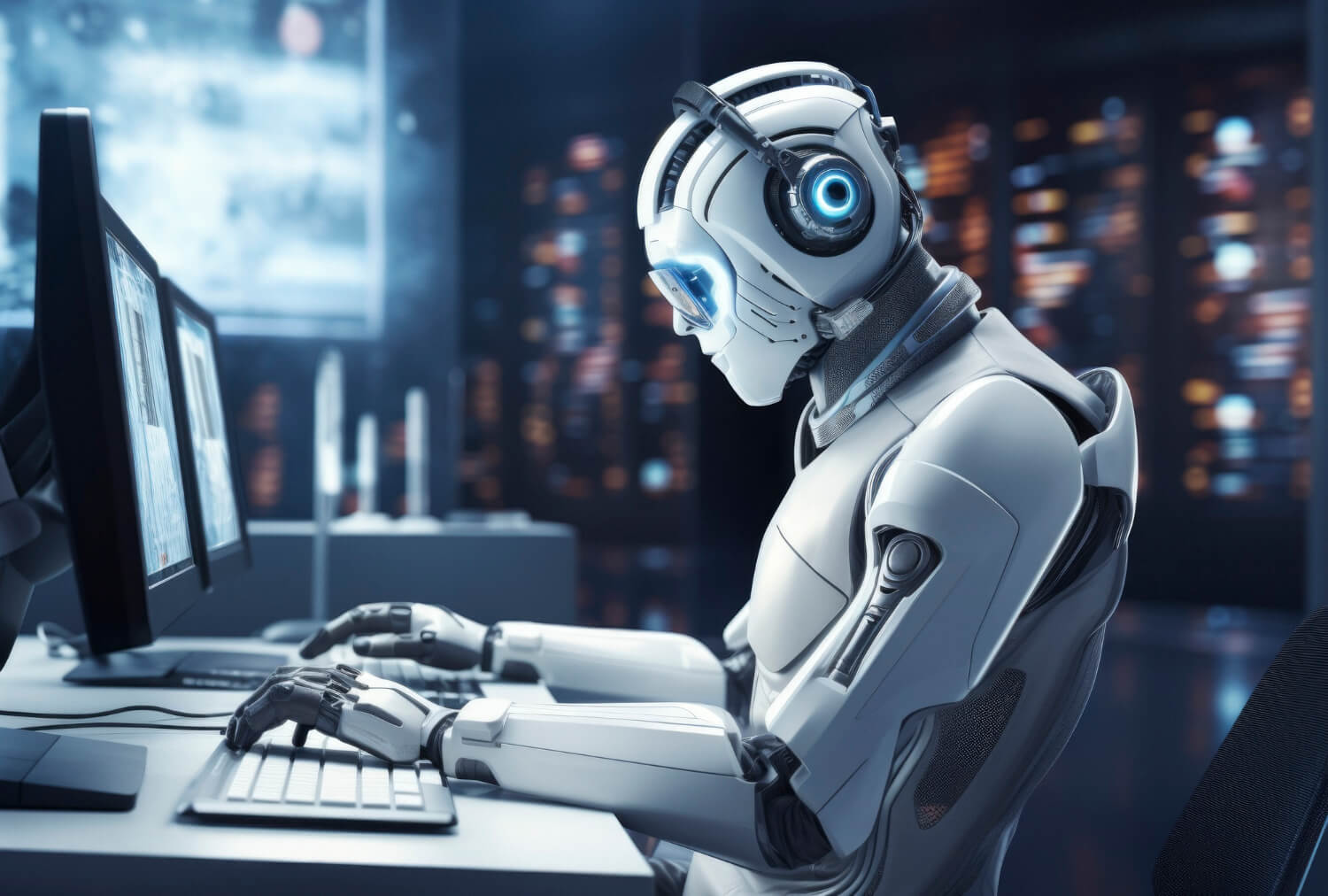
Anticipating Market Trends
Predictive analytics, a key aspect of machine learning, involves analyzing current and historical data to make predictions about future events. In affiliate marketing, this means anticipating market trends, consumer behaviors, and the effectiveness of various marketing channels.
Advanced Customer Targeting
Through machine learning, e-commerce businesses can predict which customers are most likely to respond to specific affiliate marketing campaigns. This targeted approach increases conversion rates and ensures a higher return on investment (ROI).
Dynamic Pricing and Offers
By leveraging machine learning, e-commerce businesses can dynamically adjust pricing and promotional offers based on real-time market trends and consumer behavior. This level of agility in pricing strategies can significantly impact affiliate marketing performance.
Personalized Recommendations
Machine learning algorithms can analyze customer preferences and purchasing patterns to deliver personalized product recommendations. This level of personalization enhances the affiliate marketing experience for customers, ultimately driving higher conversion rates.
Predictive Modeling for Affiliate Marketing Trends
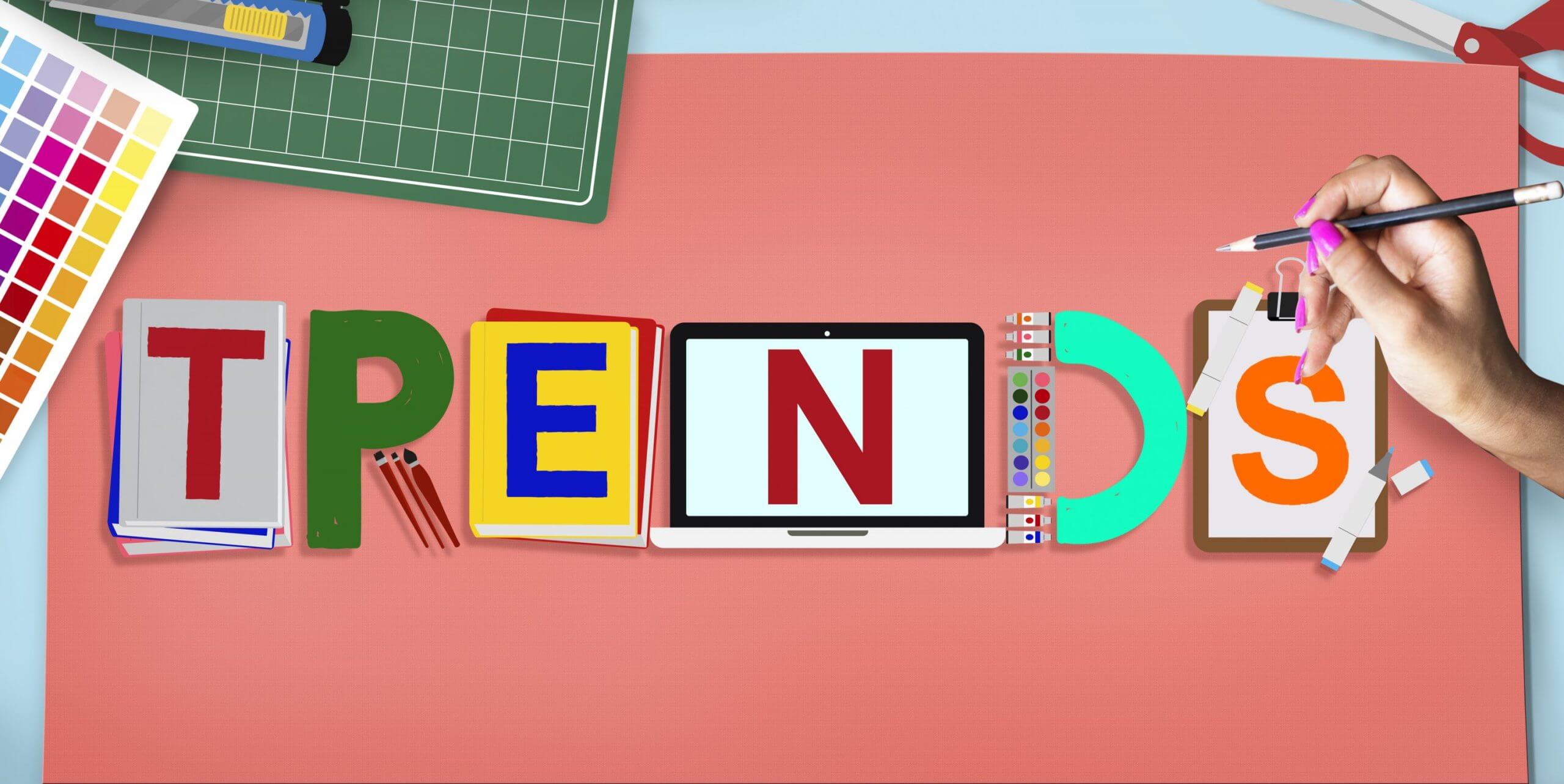
Understanding Consumer Preferences
Machine learning models can identify emerging consumer preferences and behaviors, enabling e-commerce companies to tailor their affiliate marketing strategies accordingly. This proactive approach can lead to higher engagement and conversion rates.
Forecasting Market Trends
By analyzing historical data and real-time market signals, machine learning models can forecast upcoming trends in the e-commerce landscape. Armed with this foresight, businesses can optimize their affiliate marketing strategies to capitalize on emerging opportunities.
Adapting to Seasonal Trends
Machine learning algorithms can detect seasonal patterns in consumer behavior and purchasing trends. This insight empowers e-commerce companies to adjust their affiliate marketing strategies in alignment with seasonal fluctuations, maximizing their impact during peak periods.
Conclusion
Machine learning is not just a buzzword; it’s a powerful tool that is transforming how affiliate marketing strategies are devised and executed in e-commerce. By harnessing the power of ML, e-commerce businesses can stay ahead of the curve, predicting and adapting to market trends more effectively than ever before.
Machine learning serves as a powerful ally for e-commerce businesses looking to predict affiliate marketing trends with accuracy and agility. By leveraging the capabilities of machine learning, companies can gain a competitive edge, optimize their marketing strategies, and deliver enhanced experiences to their customers in the dynamic landscape of e-commerce.
In 2023, machine learning will revolutionize e-commerce and affiliate marketing with its predictive prowess. Scaleo is your go-to affiliate marketing software for staying ahead in this dynamic landscape. It’s designed to harness the full potential of machine learning, helping you craft strategies that are not just data-driven but also future-ready. With Scaleo, you’re not just adapting your campaigns to market trends – you’re anticipating them.
Ready to give your e-commerce business the competitive edge it deserves?
Dive into the world of advanced affiliate marketing with Scaleo today. Start your free 14-days trial!
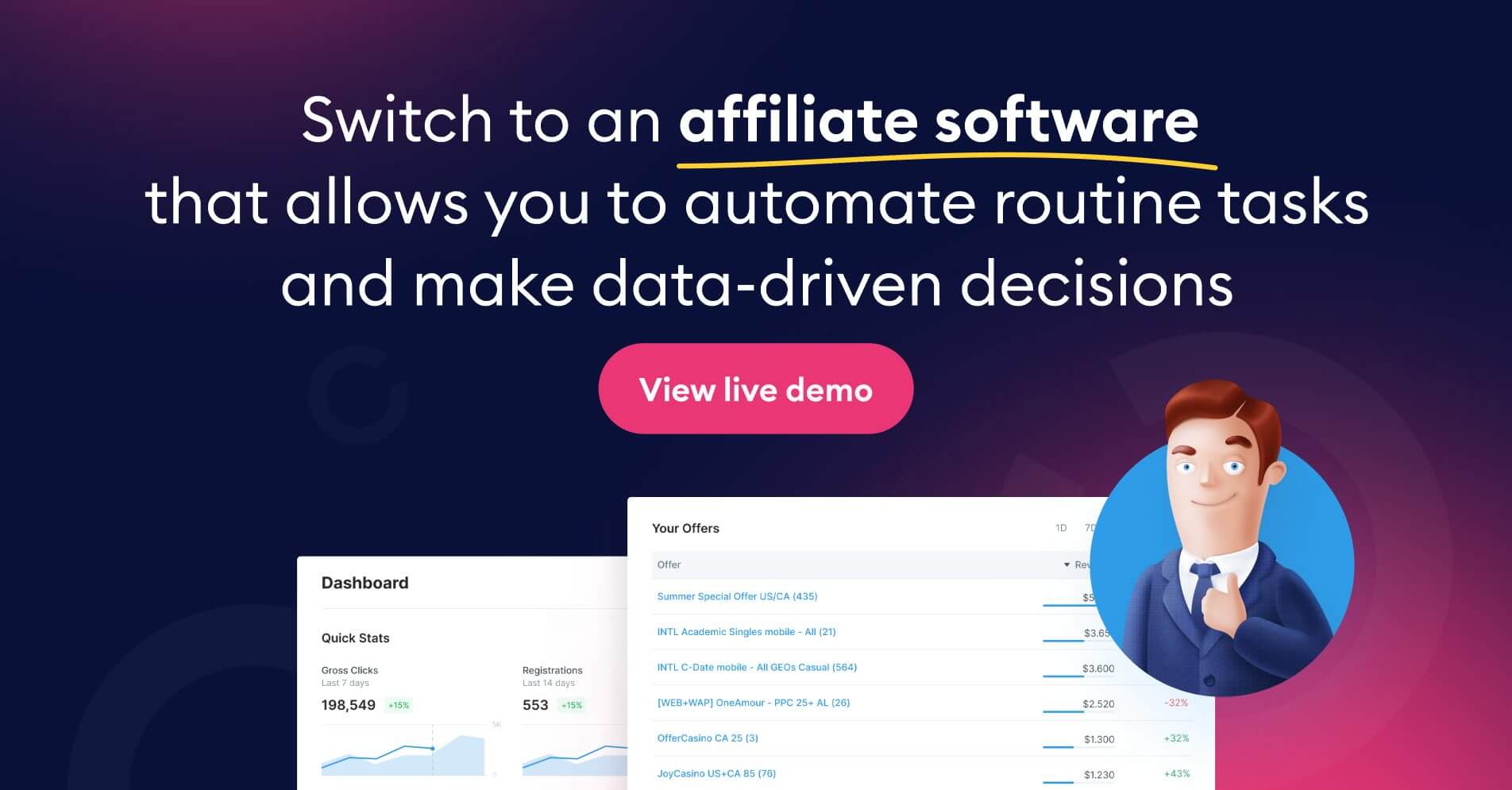
Last Updated on January 17, 2024